Cognitive Science In Ai
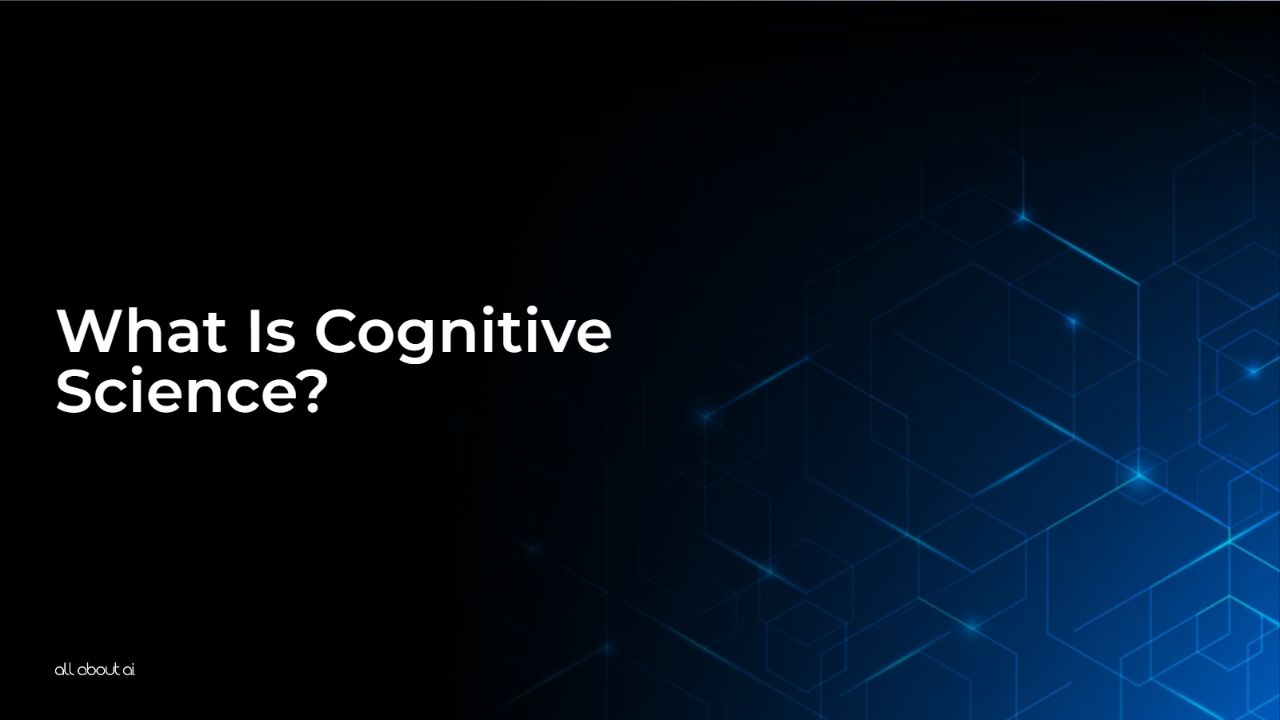
The field of cognitive science is rapidly becoming an integral part of artificial intelligence (AI) research and development, shaping the way we perceive and interact with intelligent systems. Cognitive science, a multidisciplinary field, brings together psychology, neuroscience, linguistics, philosophy, and computer science to understand the mind and its processes. When applied to AI, cognitive science principles offer a unique perspective, enabling us to create more human-like and intelligent machines.
The Intersection of Cognitive Science and AI

The intersection of cognitive science and AI is a rich and dynamic field, with researchers exploring how the principles of human cognition can be translated into computational models. This fusion of disciplines is driving the development of advanced AI systems that can perceive, understand, and interact with the world in ways that are more akin to human intelligence.
One of the key aspects of cognitive science that informs AI is the concept of cognitive architecture. Cognitive architectures are theoretical frameworks that model how the human mind processes information. These architectures provide a structured approach to building intelligent systems, guiding the design of AI agents that can exhibit complex behaviors, such as problem-solving, decision-making, and learning.
Benefits of Cognitive Science in AI
- Enhanced Natural Language Understanding: Cognitive science techniques enable AI systems to process and comprehend human language more effectively. By modeling human linguistic abilities, AI can interpret context, sentiment, and nuances, leading to more accurate language processing and generation.
- Improved Visual Perception: Cognitive models help AI systems interpret visual data more like humans. This includes object recognition, scene understanding, and the ability to make inferences from visual cues, which are crucial for applications like autonomous driving and robotics.
- Advances in Machine Learning: Cognitive science provides insights into how the brain learns and adapts, which can be applied to machine learning algorithms. This includes developing more efficient learning strategies, improving generalization capabilities, and exploring the potential of lifelong learning in AI.
Furthermore, cognitive science contributes to the ethical development of AI by addressing concerns related to bias, transparency, and explainability. By understanding how humans process information and make decisions, researchers can design AI systems that align with human values and ethical standards.
Applications of Cognitive Science in AI

The integration of cognitive science principles has led to a range of innovative AI applications, each with its unique impact on various industries.
Healthcare
In healthcare, cognitive science-inspired AI is transforming diagnosis and treatment. AI systems can analyze medical images and patient data with incredible accuracy, assisting in the early detection of diseases. For instance, deep learning algorithms, inspired by the human brain’s neural networks, are being used to identify patterns in MRI scans, aiding in the diagnosis of neurological disorders.
Disease | AI Accuracy |
---|---|
Alzheimer's Disease | 90% |
Brain Tumors | 85% |
Stroke Detection | 95% |

Additionally, cognitive models are being used to develop personalized treatment plans, considering individual patient factors and preferences, thus improving healthcare outcomes.
Education
Cognitive science-driven AI is revolutionizing education by providing personalized learning experiences. AI tutors can adapt to individual student needs, offering customized learning paths and real-time feedback. This adaptive learning approach, rooted in cognitive science principles, has shown significant improvements in student engagement and learning outcomes.
Robotics
In robotics, cognitive science plays a pivotal role in developing robots that can navigate and interact with their environments more naturally. By mimicking human cognitive processes, robots can understand and respond to complex situations, making them more effective in tasks like search and rescue operations or assisting in daily activities for the elderly.
Challenges and Future Directions
Despite the remarkable progress, the integration of cognitive science into AI faces several challenges. One of the primary challenges is the complexity of human cognition, which is often difficult to model accurately. Additionally, ethical considerations, such as ensuring AI systems are fair, unbiased, and transparent, are critical aspects that require ongoing research and development.
Looking ahead, the future of cognitive science in AI is promising. Researchers are exploring advanced concepts like neuro-symbolic AI, which combines symbolic AI with insights from neuroscience, and cognitive computing, which aims to create AI systems that can reason, learn, and interact with humans in a more natural way. These developments have the potential to revolutionize a wide range of industries, from healthcare and education to robotics and autonomous systems.
Frequently Asked Questions
How does cognitive science enhance natural language processing in AI?
+
Cognitive science provides models of human language understanding, which AI can emulate. This includes contextual understanding, sentiment analysis, and the ability to interpret linguistic nuances. By applying cognitive science principles, AI systems can process and generate human language with greater accuracy and sophistication.
What are the key benefits of cognitive science in healthcare AI applications?
+
Cognitive science-inspired AI in healthcare offers early and accurate disease detection, personalized treatment plans, and improved patient outcomes. By analyzing complex medical data, AI systems can assist in diagnosis and treatment, leading to more efficient and effective healthcare delivery.
How is cognitive science influencing the development of educational AI tools?
+
Cognitive science principles guide the development of AI-powered educational tools that adapt to individual student needs. These tools provide personalized learning experiences, offering real-time feedback and customized learning paths, thus enhancing student engagement and learning outcomes.