Skip.
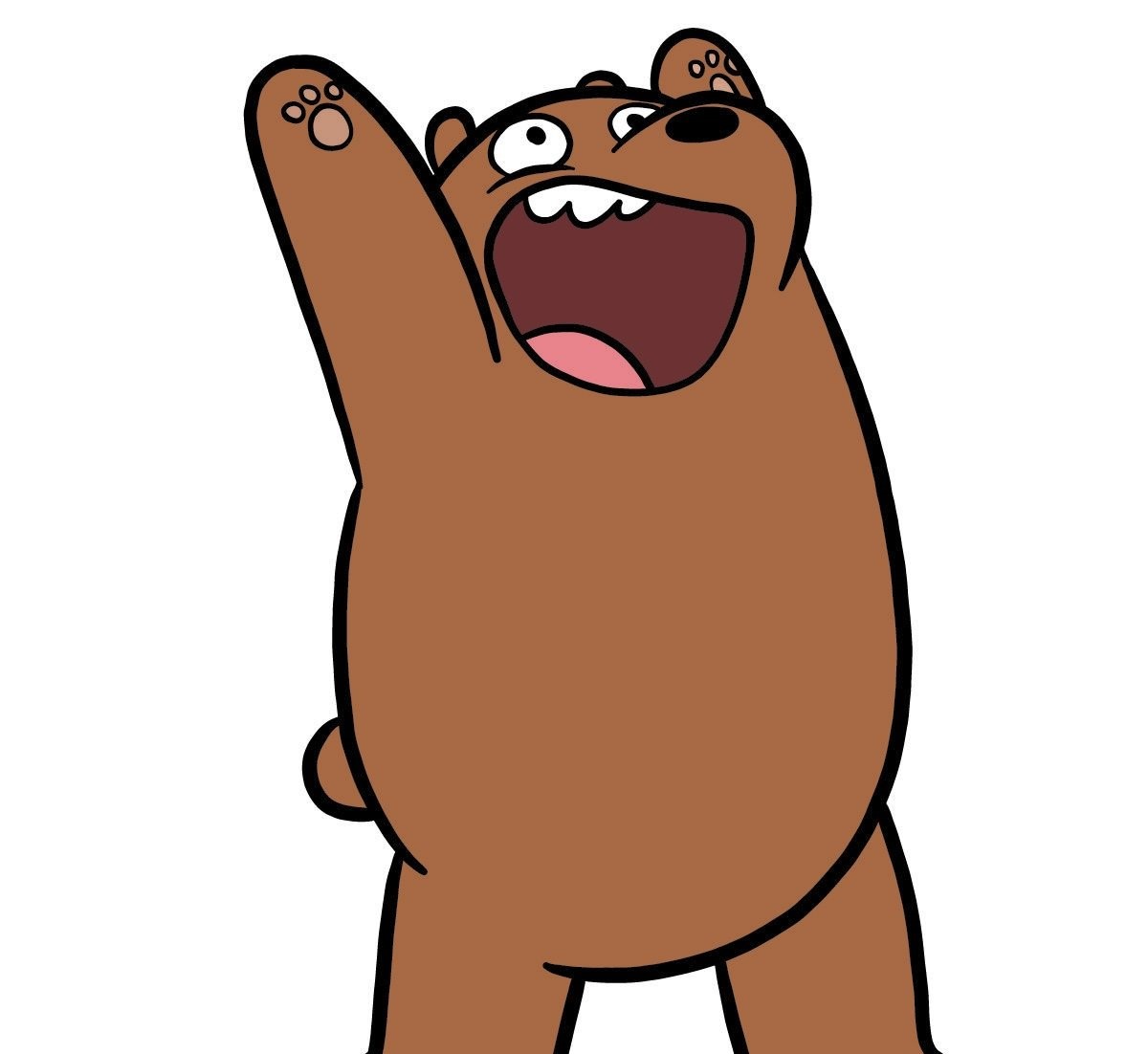
The rapidly evolving world of AI and machine learning has seen a surge in the development of large language models (LLMs), which have the potential to revolutionize various industries and enhance our daily lives. These models, with their impressive capabilities, are designed to understand and generate human-like text, opening up a myriad of applications across different sectors. However, with the immense power these models possess, there is an equal need for ethical considerations and responsible development practices to ensure their benefits are realized without causing unintended harm.
The Rise of Large Language Models

Large Language Models have gained significant attention and momentum in recent years, thanks to their ability to process and generate natural language with remarkable accuracy. These models, trained on vast amounts of text data, can comprehend and produce coherent, contextually relevant text, making them invaluable for tasks such as language translation, text summarization, content generation, and even providing sophisticated conversational interfaces.
One of the most notable LLMs, GPT-3 (Generative Pre-trained Transformer 3), developed by OpenAI, has demonstrated groundbreaking performance in various natural language processing tasks. With its 175 billion parameters, GPT-3 has shown an impressive ability to generate human-like text, understand complex language nuances, and perform tasks that were previously thought to be beyond the reach of AI. This has opened up new possibilities for developers and businesses, offering innovative solutions to enhance user experiences and operational efficiency.
Other notable LLMs include Microsoft's Turing NLG, which has shown exceptional performance in language understanding and generation tasks, and Google's BERT (Bidirectional Encoder Representations from Transformers), which has significantly improved language understanding in tasks like sentiment analysis and question answering. These models, along with many others, are pushing the boundaries of what's possible with natural language processing, driving innovation and competition in the AI space.
Key Advantages of LLMs
Large Language Models offer several key advantages that make them invaluable in various applications:
- Human-like Text Generation: LLMs can generate text that is incredibly similar to that produced by humans, making them ideal for content creation, creative writing, and even generating personalized responses in customer support scenarios.
- Contextual Understanding: These models have an advanced understanding of language context, enabling them to grasp the nuances of different languages and generate responses that are relevant and coherent.
- Scalability: LLMs can process vast amounts of data quickly and efficiently, making them scalable for large-scale applications and businesses.
- Versatility: With their ability to handle various natural language tasks, LLMs offer versatility, allowing them to be applied across different industries and use cases.
However, with these advantages come significant challenges and considerations that must be addressed to ensure the responsible and ethical development and deployment of LLMs.
Ethical Considerations in LLM Development

The development and deployment of Large Language Models present a myriad of ethical challenges that need careful consideration to ensure these powerful tools are used responsibly and for the benefit of society as a whole. Here are some key ethical considerations that developers, researchers, and organizations should keep in mind:
Bias and Fairness
LLMs, like any AI model, are susceptible to bias, which can be inadvertently learned from the data they are trained on. Bias in language models can manifest as unfair or discriminatory outputs, reinforcing stereotypes or marginalizing certain groups. For instance, a language model trained on historical data might reflect and perpetuate societal biases, leading to unfair or inaccurate predictions or suggestions.
To address this, developers should ensure that training data is diverse, representative, and free from biases. Regular audits and evaluations of the model's output can help identify and mitigate biases. Additionally, involving diverse teams in the development process can provide different perspectives and help identify potential biases that might otherwise be overlooked.
Type of Bias | Potential Impact |
---|---|
Gender Bias | Discriminatory language or gender-stereotypical outputs. |
Racial Bias | Reinforcement of racial stereotypes or inaccurate representations. |
Political Bias | Biased recommendations or suggestions favoring certain political ideologies. |

Privacy and Data Protection
Large Language Models often require vast amounts of data for training, which can include sensitive or personal information. Ensuring the privacy and protection of this data is crucial to maintaining user trust and compliance with data protection regulations like GDPR or CCPA.
Developers should implement robust data protection measures, such as anonymization techniques, secure data storage, and strict access controls. Additionally, being transparent about data collection and usage practices can help build trust with users and ensure compliance with privacy regulations.
Accountability and Transparency
With the increasing complexity and impact of Large Language Models, ensuring accountability and transparency in their development and deployment becomes essential. This includes being transparent about the model’s capabilities, limitations, and potential biases, as well as having clear processes for addressing concerns or issues that may arise.
Developers should strive to be transparent about the data used to train the model, the model's architecture, and the evaluation metrics employed. Providing clear and accessible documentation can help users and other stakeholders understand the model's behavior and make informed decisions about its use.
Impact on Employment and Society
The widespread adoption of Large Language Models has the potential to significantly impact employment across various sectors. While these models can automate certain tasks, leading to increased efficiency, they may also displace certain jobs or require workers to upskill or reskill. Developers and organizations should consider the societal impact of their models and work towards minimizing negative consequences and promoting inclusive growth.
Additionally, the integration of LLMs into various aspects of daily life, from education to healthcare, requires careful consideration of the potential social implications. Ensuring that these models are used ethically and responsibly can help mitigate potential harms and promote a fair and just society.
Responsible Development and Deployment
Ensuring the responsible development and deployment of Large Language Models is crucial to maximizing their benefits while minimizing potential harms. Here are some key practices that can help promote responsible AI development and deployment:
Diverse Development Teams
Involving diverse teams in the development process can help identify and mitigate potential biases. A diverse team brings different perspectives, experiences, and cultural understandings, which can lead to more inclusive and unbiased models. Additionally, diverse teams can provide valuable insights into potential societal impacts, helping to shape the model’s development and deployment in a way that benefits a broader range of users.
Regular Audits and Evaluations
Regular audits and evaluations of the model’s performance and outputs can help identify and address issues related to bias, fairness, and accuracy. These audits should be comprehensive, covering various aspects of the model’s behavior, including its performance on different demographics, its handling of sensitive topics, and its adherence to ethical guidelines.
Clear Documentation and Communication
Providing clear and accessible documentation about the model’s capabilities, limitations, and potential biases is essential for promoting transparency and trust. This documentation should be written in plain language and made easily accessible to users, researchers, and other stakeholders. Additionally, developers should actively communicate with users and the wider community, addressing concerns and providing updates on the model’s development and performance.
Collaborative Governance and Regulation
The development and deployment of Large Language Models should be guided by collaborative governance and regulation. This involves working closely with policymakers, researchers, industry experts, and civil society organizations to establish ethical guidelines and best practices. By engaging in open dialogue and collaboration, developers can ensure that their models are developed and deployed in a manner that aligns with societal values and ethical standards.
Continued Research and Improvement
The field of Large Language Models is rapidly evolving, and ongoing research is essential to keep up with the latest advancements and challenges. Developers should actively engage in research and development to improve their models, address emerging issues, and stay informed about best practices in ethical AI development. This continuous improvement process ensures that models remain effective, efficient, and ethically sound over time.
The Future of Large Language Models
The future of Large Language Models holds immense potential, with ongoing advancements and research pushing the boundaries of what these models can achieve. As we continue to explore and refine these powerful tools, several key trends and developments are likely to shape the future of LLMs:
Continued Advancements in Model Architecture
Researchers are continuously working on improving the architecture of LLMs, aiming to enhance their capabilities and efficiency. This includes developing more sophisticated transformer-based models, exploring new neural network architectures, and optimizing training techniques to improve performance and reduce computational requirements.
One notable trend is the exploration of multi-modal LLMs, which can understand and generate not just text but also images, audio, and other forms of data. These models have the potential to revolutionize fields like computer vision and natural language understanding, enabling more holistic and contextually aware AI systems.
Enhanced Data Efficiency
Training Large Language Models often requires vast amounts of data, which can be challenging to collect, store, and process. To address this, researchers are focusing on developing models that are more data-efficient, requiring less training data to achieve high performance. This includes exploring techniques like transfer learning, where pre-trained models are fine-tuned for specific tasks, and meta-learning, which enables models to learn from fewer examples.
Ethical and Responsible Development
As discussed earlier, the ethical considerations surrounding LLMs are of utmost importance. The future of these models will likely be shaped by increased focus on responsible development and deployment practices. This includes continuing to address biases, ensuring privacy and data protection, and promoting transparency and accountability.
Additionally, there is growing interest in developing LLMs that are explainable and interpretable, allowing users and developers to understand how the model arrives at its decisions and outputs. This not only enhances trust and transparency but also enables better control and management of these powerful models.
Integration into Daily Life
Large Language Models are already being integrated into various aspects of our daily lives, from virtual assistants and chatbots to content generation and personalized recommendations. As these models continue to improve, we can expect even more seamless and intuitive interactions with AI-powered systems. This includes more sophisticated language understanding, enabling more natural and human-like conversations, and the ability to handle complex tasks and queries.
Furthermore, LLMs have the potential to revolutionize industries such as healthcare, education, and finance, offering personalized solutions and improving efficiency. However, this integration requires careful consideration of ethical and societal implications to ensure these models are used for the benefit of all.
Collaborative and Open-Source Development
The development of Large Language Models often involves substantial resources and expertise. To address this, there is a growing trend towards collaborative and open-source development, where researchers and developers from different organizations and backgrounds come together to share knowledge, resources, and best practices. This collaborative approach not only accelerates development but also promotes diversity and inclusivity, leading to more robust and ethically sound models.
Frequently Asked Questions
How do Large Language Models work?
+Large Language Models are based on advanced neural network architectures, particularly transformer-based models like GPT-3. These models are trained on vast amounts of text data, learning to predict the next word in a sequence based on the surrounding context. This enables them to generate human-like text and understand complex language nuances.
What are some common applications of LLMs?
+LLMs have a wide range of applications, including language translation, text summarization, content generation, chatbots, virtual assistants, sentiment analysis, question answering, and more. They are also being explored for use in healthcare, education, and finance, offering personalized solutions and improving efficiency.
What are the key challenges in developing LLMs?
+Developing LLMs comes with several challenges, including the need for vast amounts of high-quality training data, computational resources, and expertise. Additionally, ensuring ethical considerations like bias mitigation, privacy protection, and accountability is crucial to responsible development.
How can we ensure the responsible development and deployment of LLMs?
+Ensuring responsible development and deployment of LLMs involves a multi-faceted approach. This includes involving diverse development teams, conducting regular audits and evaluations, providing clear documentation and communication, collaborating with stakeholders for governance and regulation, and continuously researching and improving the models.
What does the future hold for Large Language Models?
+The future of LLMs is promising, with ongoing advancements in model architecture, data efficiency, and ethical considerations. We can expect more sophisticated models, better integration into daily life, and continued collaborative and open-source development. However, addressing ethical and societal implications will remain a key focus to ensure these models benefit society as a whole.