The Top 3 Secrets Unveiled
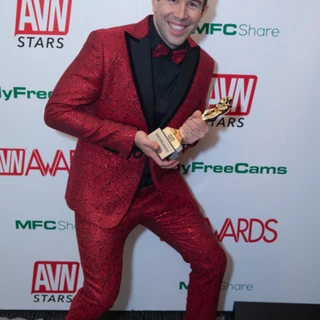

Unlocking the Power of Advanced AI Tools: A Comprehensive Guide

In the rapidly evolving landscape of artificial intelligence, the potential of advanced AI tools is limitless. As technology continues to advance, so does the need for comprehensive understanding and utilization of these powerful resources. This article delves into the top three secrets of advanced AI tools, revealing the transformative capabilities that can propel businesses and individuals into the future.
By harnessing the power of AI, organizations can gain a competitive edge, streamline processes, and revolutionize their industries. From automated decision-making to predictive analytics, the possibilities are vast. However, to fully unlock the potential of AI, one must delve into the intricacies of these tools and discover the hidden gems within.
In this comprehensive guide, we will explore the secrets that lie beneath the surface of advanced AI tools, shedding light on their capabilities, applications, and impact. Get ready to embark on a journey of discovery, where the boundaries of artificial intelligence will be pushed, and the future of innovation will be unveiled.
Chapter 1: The Power of Natural Language Processing (NLP)
Natural Language Processing, often referred to as NLP, is a branch of AI that focuses on the interaction between computers and human language. It is a powerful tool that enables machines to understand, interpret, and generate human language, opening up a world of possibilities.
1.1 Understanding the Human Language
At its core, NLP aims to bridge the gap between human communication and machine understanding. By employing advanced algorithms and linguistic models, NLP tools can analyze and process vast amounts of text data, uncovering valuable insights and patterns.
One of the key strengths of NLP lies in its ability to comprehend the nuances of language, including context, sentiment, and intent. This enables machines to interpret not just the words themselves but the underlying meaning and emotion behind them.
NLP Application | Description |
---|---|
Sentiment Analysis | Identifying the sentiment or emotion expressed in a text, whether positive, negative, or neutral. |
Named Entity Recognition | Extracting and classifying named entities such as people, organizations, and locations from text. |
Text Classification | Categorizing text into predefined classes or topics based on its content. |

These capabilities have far-reaching applications, from improving customer support through sentiment analysis to enhancing information retrieval systems with more accurate text classification.
1.2 Real-World Applications of NLP
The power of NLP is evident in its diverse range of real-world applications. Here are some notable examples:
- Virtual Assistants: NLP powers virtual assistants like Siri, Alexa, and Google Assistant, enabling them to understand and respond to user queries and commands.
- Language Translation: NLP algorithms are at the heart of machine translation services, allowing for accurate and efficient language conversion.
- Content Moderation: Social media platforms and online communities utilize NLP to detect and filter inappropriate or harmful content.
- Text Summarization: NLP techniques can automatically generate concise summaries of lengthy texts, saving time and effort.
- Chatbots: Many businesses employ chatbots powered by NLP to provide instant customer support and enhance user experiences.
The impact of NLP extends beyond these examples, with applications in healthcare, finance, legal services, and more. By leveraging NLP, organizations can gain deeper insights from text data, improve decision-making, and enhance user interactions.
Chapter 2: Unlocking the Potential of Machine Learning
Machine Learning (ML) is a subset of AI that focuses on the development of algorithms and models that can learn and improve over time, based on data and experience. It is a powerful tool that enables AI systems to adapt, evolve, and make predictions or decisions without explicit programming.
2.1 The Fundamentals of Machine Learning
At its core, ML involves training models using large datasets to recognize patterns and make informed predictions or decisions. This process of learning from data is what sets ML apart from traditional rule-based systems.
There are three main types of ML algorithms:
- Supervised Learning: Models are trained on labeled data, where the correct output is provided for each input. This allows the model to learn the mapping between inputs and outputs.
- Unsupervised Learning: Models are trained on unlabeled data, where the goal is to find patterns or structures in the data without any predefined labels.
- Reinforcement Learning: Models learn through trial and error, receiving feedback in the form of rewards or penalties based on their actions.
2.2 Real-World Applications of Machine Learning
Machine Learning has revolutionized numerous industries and applications. Here are some notable examples:
- Image Recognition: ML algorithms can identify and classify objects, faces, and scenes in images and videos, with applications in security, healthcare, and autonomous vehicles.
- Recommendation Systems: Online platforms use ML to suggest products, services, or content based on user preferences and behavior, enhancing user experiences.
- Fraud Detection: ML models can analyze patterns and detect anomalies in financial transactions, helping to identify and prevent fraudulent activities.
- Predictive Maintenance: In industrial settings, ML is used to predict equipment failures and optimize maintenance schedules, reducing downtime and costs.
- Natural Language Processing: As mentioned earlier, ML plays a crucial role in NLP, enabling systems to understand and generate human language.
The impact of Machine Learning extends across various domains, from healthcare and finance to transportation and entertainment. By harnessing the power of ML, organizations can make data-driven decisions, automate processes, and deliver personalized experiences.
Chapter 3: The Future of AI: Generative Models and Beyond
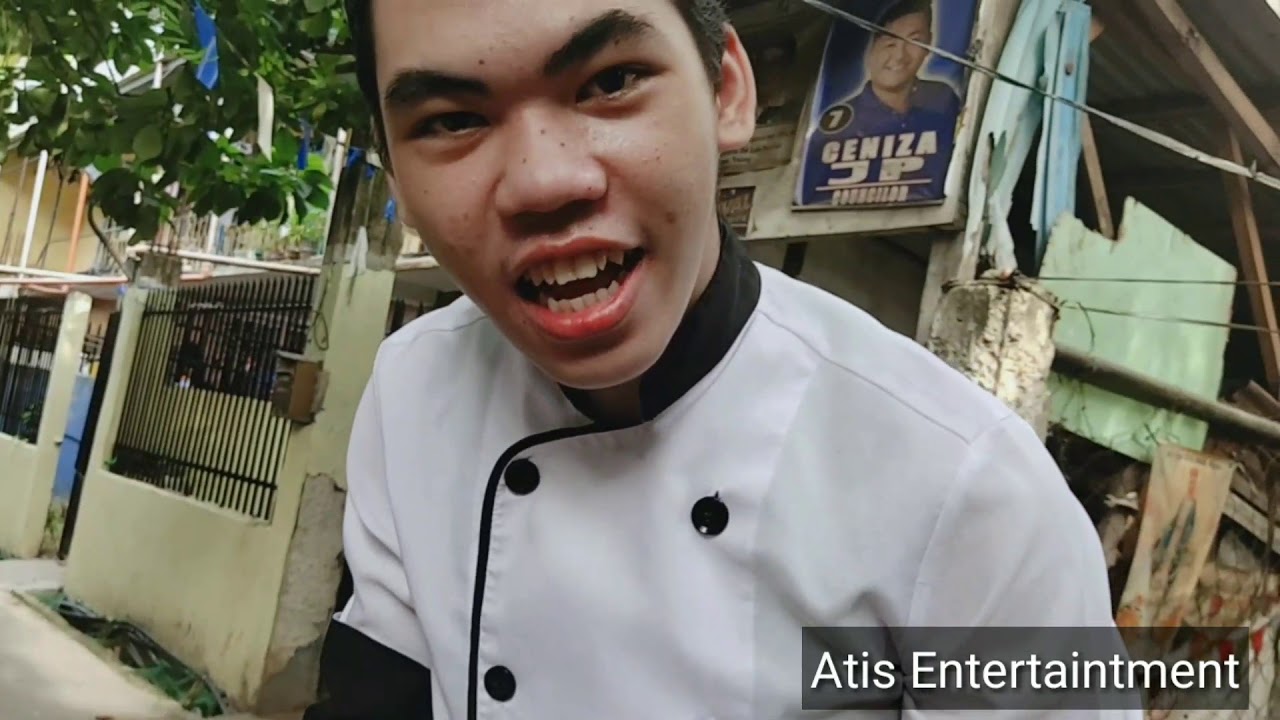
As AI continues to evolve, the development of generative models is pushing the boundaries of what is possible. Generative models are a type of AI algorithm that can generate new data, such as images, text, or even music, based on patterns learned from existing data.
3.1 Understanding Generative Models
Generative models are a subset of Machine Learning that focuses on creating new data rather than just making predictions or classifications. These models learn the underlying structure and distribution of the training data and use that knowledge to generate new, synthetic data.
There are various types of generative models, each with its own strengths and applications:
- Generative Adversarial Networks (GANs): GANs consist of two neural networks, a generator, and a discriminator, that compete against each other to generate increasingly realistic data.
- Variational Autoencoders (VAEs): VAEs are a type of neural network that learns to encode input data into a latent space and then generate new data by decoding from this space.
- Normalizing Flows: Normalizing flows are a class of generative models that use a series of invertible transformations to map data from a simple distribution to a complex one.
3.2 Real-World Applications of Generative Models
Generative models have the potential to revolutionize various industries and applications. Here are some notable examples:
- Image Synthesis: Generative models can create realistic images, including faces, landscapes, and even artistic styles, with applications in entertainment, advertising, and content creation.
- Text Generation: These models can generate coherent and contextually relevant text, with potential uses in content generation, chatbots, and language translation.
- Music Composition: Generative models can compose new music based on learned patterns, offering a new approach to music creation and experimentation.
- Healthcare: In the medical field, generative models can assist in drug discovery, disease diagnosis, and personalized treatment planning.
- Data Augmentation: Generative models can be used to augment existing datasets, helping to improve the performance of other AI models, especially in data-scarce scenarios.
The future of AI is bright, and generative models are at the forefront of this evolution. By harnessing the power of these advanced tools, organizations can unlock new possibilities, drive innovation, and shape the future of technology.
Conclusion: Unlocking the Full Potential
In this comprehensive guide, we have unveiled the top three secrets of advanced AI tools: Natural Language Processing, Machine Learning, and Generative Models. By understanding and harnessing the power of these technologies, businesses and individuals can unlock a world of possibilities.
From automating tasks and improving decision-making to creating new forms of content and driving innovation, AI has the potential to transform industries and shape the future. As we continue to explore and develop these advanced tools, the possibilities for growth and advancement are limitless.
As we conclude this journey, remember that the secrets unveiled here are just the beginning. The true potential of AI lies in the hands of those who dare to explore, innovate, and push the boundaries of what is possible. Embrace the power of advanced AI tools, and let them empower you to achieve your goals and create a brighter future.
What are some potential ethical concerns surrounding advanced AI tools?
+
Advanced AI tools, while powerful, come with ethical considerations. Bias in data and algorithms can lead to unfair decisions or discriminatory outcomes. Privacy and data protection are also crucial, as AI often relies on large amounts of personal data. Responsible development and deployment, with a focus on transparency and accountability, are essential to mitigating these concerns.
How can businesses leverage advanced AI tools for competitive advantage?
+
Businesses can gain a competitive edge by utilizing advanced AI tools for various purposes. This includes automating processes, improving decision-making through data analysis, and delivering personalized experiences to customers. By staying at the forefront of AI innovation, businesses can stay ahead of the competition and drive growth.
What are some challenges in implementing advanced AI tools?
+
Implementing advanced AI tools can present challenges such as data quality and availability, model training and optimization, and the need for specialized expertise. Additionally, ethical considerations and ensuring the responsible use of AI are crucial aspects to address during implementation.